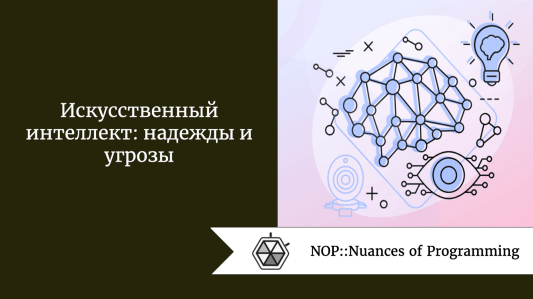
Artificial intelligence does not follow the logic of scientific progress
We have written many times in MT about researchers and professionals who proclaim machine learning systems as “black boxes” (1) even for those who build them. This makes it difficult to evaluate results and reuse emerging algorithms.
Neural networks - the technique that gives us intelligent converting bots and ingenious text generators that can even create poetry - remains an incomprehensible mystery to outside observers.
They are getting bigger and more complex, handling huge datasets, and using massive compute arrays. This makes replication and analysis of the obtained models costly and sometimes impossible for other researchers, except for large centers with huge budgets.
Many scientists are well aware of this problem. Among them is Joel Pino (2), chairman of NeurIPS, the premier conference on reproducibility. The experts under her leadership want to create a "reproducibility checklist".
The idea, Pino said, is to encourage researchers to offer others a roadmap so they can recreate and use the work already done. You can marvel at the eloquence of a new text generator or the superhuman dexterity of a video game robot, but even the best experts have no idea how these wonders work. Therefore, the reproduction of AI models is important not only for identifying new goals and directions for research, but also as a purely practical guide to use.
Others are trying to solve this problem. Google researchers offered "model cards" to describe in detail how the systems were tested, including results that point to potential bugs. Researchers at the Allen Institute for Artificial Intelligence (AI2) have published a paper that aims to extend the Pinot reproducibility checklist to other steps in the experimental process. “Show your work,” they urge.
Sometimes basic information is missing because the research project is owned, especially by laboratories working for the company. More often, however, it is a sign of an inability to describe changing and increasingly complex research methods. Neural networks are a very complex area. To get the best results, fine tuning of thousands of "knobs and buttons" is often required, which some call "black magic". The choice of the optimal model is often associated with a large number of experiments. Magic becomes very expensive.
For example, when Facebook tried to replicate the work of AlphaGo, a system developed by DeepMind Alphabet, the task proved extremely difficult. Huge computational requirements, millions of experiments on thousands of devices over many days, combined with the lack of code, made the system "very difficult, if not impossible, to recreate, test, improve, and extend," according to Facebook employees.
The problem seems to be specialized. However, if we think further, the phenomenon of problems with the reproducibility of results and functions between one research team and another undermines all the logic of the functioning of science and research processes known to us. As a rule, the results of previous research can be used as a basis for further research that stimulates the development of knowledge, technology and general progress.
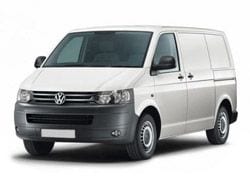
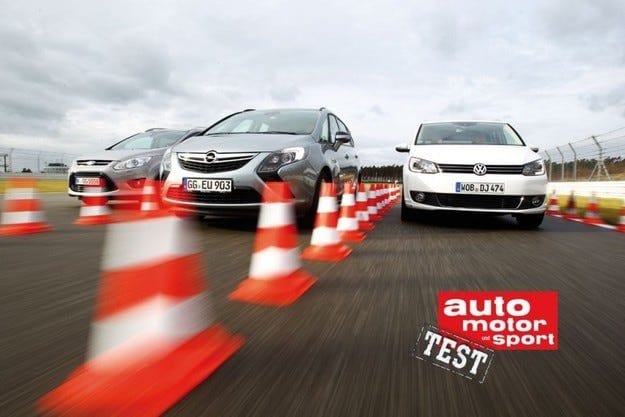