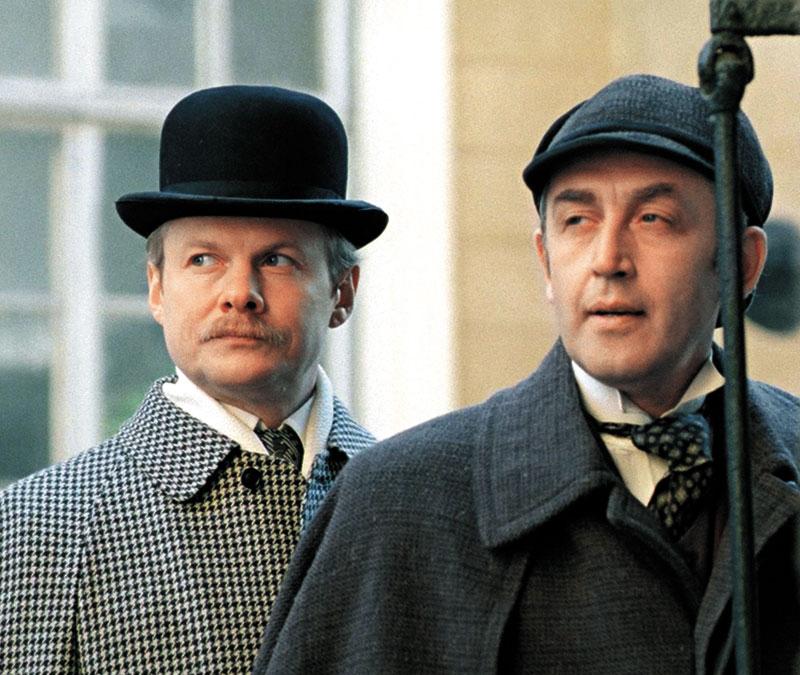
Watson did not bite the doctor, and very well
Content
Although, as in many other fields, the enthusiasm to replace doctors with AI has waned somewhat after a series of diagnostic failures, work on the development of AI-based medicine is still ongoing. Because, nevertheless, they still offer great opportunities and a chance to improve the efficiency of operations in many of its areas.
IBM was announced in 2015, and in 2016 it gained access to data from four major patient data companies (1). The most famous, thanks to numerous media reports, and at the same time the most ambitious project using advanced artificial intelligence from IBM was related to oncology. Scientists have been trying to use the vast resources of data to process them in order to turn them into well-adapted anti-cancer therapies. Long term goal was to get Watson to referee clinical trials and results as a doctor would.
1. One of the visualizations of the Watson Health medical system
However, it turned out that Watson cannot independently refer to medical literature, and also cannot extract information from electronic medical records of patients. However, the most serious accusation against him was that inability to effectively compare a new patient with other older cancer patients and detect symptoms that are invisible at first glance.
There were, admittedly, some oncologists who claimed to have confidence in his judgment, albeit mostly in terms of Watson's suggestions for standard treatments, or as an additional, additional medical opinion. Many have pointed out that this system will be a great automated librarian for physicians.
As a result of not very flattering reviews from IBM problems with the sale of the Watson system in US medical institutions. IBM sales representatives managed to sell it to some hospitals in India, South Korea, Thailand and other countries. In India, doctors () evaluated Watson's recommendations for 638 cases of breast cancer. The compliance rate for treatment recommendations is 73%. Worse Watson dropped out at the Gachon Medical Center in South Korea, where his best recommendations for 656 colorectal cancer patients matched expert recommendations only 49 percent of the time. Doctors have assessed that Watson didn't do well with older patientsby not offering them certain standard medicines, and made the critical mistake of undertaking aggressive treatment surveillance for some patients with metastatic disease.
Ultimately, although his work as a diagnostician and physician is considered unsuccessful, there are areas in which he has proved extremely useful. Product Watson for Genomics, which was developed in collaboration with the University of North Carolina, Yale University, and other institutions, is used genetic laboratories for preparing reports for oncologists. Watson downloads list file genetic mutations in a patient and can generate a report in minutes that includes suggestions for all important drugs and clinical trials. Watson handles genetic information with relative easebecause they are presented in structured files and do not contain ambiguities - either there is a mutation or there is no mutation.
IBM partners at the University of North Carolina published a paper on efficiency in 2017. Watson found potentially important mutations that had not been identified by human studies in 32% of them. patients studied, making them good candidates for the new drug. However, there is still no evidence that use leads to better treatment outcomes.
Domestication of proteins
This and many other examples contribute to the growing belief that all deficiencies in health care are being addressed, but we need to look for areas where this can really help, because people are not doing very well there. Such a field is, for example, protein research. Last year, information emerged that it could accurately predict the shape of proteins based on their sequence (2). This is a traditional task, beyond the power of not only people, but even powerful computers. If we master the precise modeling of the twisting of protein molecules, there will be huge opportunities for gene therapy. Scientists hope that with the help of AlphaFold we will study the functions of thousands, and this, in turn, will allow us to understand the causes of many diseases.
Figure 2. Protein twisting modeled with DeepMind's AlphaFold.
Now we know two hundred million proteins, but we fully understand the structure and function of a small part of them. Proteins it is the basic building block of living organisms. They are responsible for most of the processes occurring in cells. How they work and what they do is determined by their 50D structure. They take the appropriate form without any instructions, guided by the laws of physics. For decades, experimental methods have been the main method for determining the shape of proteins. In the XNUMXs, the use X-ray crystallographic methods. In the last decade, it has become the research tool of choice. crystal microscopy. In the 80s and 90s, work began on using computers to determine the shape of proteins. However, the results still did not satisfy the scientists. Methods that worked for some proteins did not work for others.
Already in 2018 Alpha Fold received recognition from experts in protein modeling. However, at the time it used methods very similar to other programs. The scientists changed tactics and created another, which also used information about the physical and geometric restrictions in the folding of protein molecules. Alpha Fold gave uneven results. Sometimes he did better, sometimes worse. But almost two-thirds of his predictions coincided with the results obtained by experimental methods. At the beginning of year 2, the algorithm described the structure of several proteins of the SARS-CoV-3 virus. Later, it was found that the predictions for the Orf2020a protein are consistent with the results obtained experimentally.
It is not only about studying the internal ways of folding proteins, but also about design. Researchers from the NIH BRAIN initiative used machine learning develop a protein that can track brain serotonin levels in real time. Serotonin is a neurochemical that plays a key role in how the brain controls our thoughts and feelings. For example, many antidepressants are designed to change the serotonin signals that are transmitted between neurons. In an article in the journal Cell, scientists described how they use advanced genetic engineering methods turn a bacterial protein into a new research tool that could help track serotonin transmission with greater accuracy than current methods. Preclinical experiments, mostly in mice, have shown that the sensor can instantly detect subtle changes in brain serotonin levels during sleep, fear and social interactions, and test the effectiveness of new psychoactive drugs.
The fight against the pandemic has not always been successful
After all, this was the first epidemic we wrote about in MT. However, for example, if we talk about the very process of the development of the pandemic, then at the initial stage, AI seemed to be something of a failure. Scholars have complained that Artificial Intelligence cannot correctly predict the extent of the spread of coronavirus based on data from previous epidemics. “These solutions work well in some areas, such as recognizing faces that have a certain number of eyes and ears. SARS-CoV-2 epidemic These are previously unknown events and many new variables, so artificial intelligence based on the historical data that was used to train it does not work well. The pandemic has shown that we need to look for other technologies and approaches,” Maxim Fedorov from Skoltech said in an April 2020 statement to the Russian media.
Over time there were however algorithms that seem to prove the great usefulness of AI in the fight against COVID-19. Scientists in the US developed a system in the fall of 2020 to recognize characteristic cough patterns in people with COVID-19, even if they had no other symptoms.
When vaccines appeared, the idea was born to help vaccinate the population. She could, for example help model transportation and logistics of vaccines. Also in determining which populations should be vaccinated first to deal with the pandemic faster. It would also help forecast demand and optimize the timing and speed of vaccination by quickly identifying problems and bottlenecks in logistics. The combination of algorithms with constant monitoring can also provide information quickly about possible side effects and health events.
these systems using AI in optimizing and improving healthcare are already known. Their practical advantages were appreciated; for example, the health care system developed by Macro-Eyes at Stanford University in the US. As is the case with many other medical institutions, the problem was the lack of patients who did not show up for appointments. Macro Eyes built a system that could reliably predict which patients were not likely to be there. In some situations, he could also suggest alternative times and locations for clinics, which would increase the chances of a patient showing up. Later, similar technology was applied in various places from Arkansas to Nigeria with support, in particular, the US Agency for International Development i.
In Tanzania, Macro-Eyes worked on a project aimed at increasing child immunization rates. The software analyzed how many doses of vaccines needed to be sent to a given vaccination center. He was also able to assess which families might be reluctant to vaccinate their children, but they could be persuaded with appropriate arguments and the location of a vaccination center in a convenient location. Using this software, the Tanzanian government has been able to increase the effectiveness of its immunization program by 96%. and reduce vaccine waste to 2,42 per 100 people.
In Sierra Leone, where residents' health data was missing, the company tried to match this with information about education. It turned out that the number of teachers and their students alone was enough to predict 70 percent. the accuracy of whether the local clinic has access to clean water, which is already a footprint of data on the health of the people living there (3).
3. Macro-Eyes illustration of AI-driven healthcare programs in Africa.
The myth of the machine doctor does not disappear
Despite failures Watson new diagnostic approaches are still being developed and are considered to be more and more advanced. Comparison made in Sweden in September 2020. used in imaging diagnostics of breast cancer showed that the best of them works in the same way as a radiologist. The algorithms have been tested using nearly nine thousand mammography images obtained during routine screening. Three systems, designated as AI-1, AI-2 and AI-3, achieved an accuracy of 81,9%, 67%. and 67,4%. For comparison, for radiologists who interpret these images as the first, this figure was 77,4%, and in the case of radiologistswho was the second to describe it, it was 80,1 percent. The best of the algorithms was also able to detect cases that radiologists missed during screening, and women were diagnosed as sick in less than a year.
According to the researchers, these results prove that artificial intelligence algorithms help correct false-negative diagnoses made by radiologists. Combining the capabilities of AI-1 with an average radiologist increased the number of detected breast cancers by 8%. The team at the Royal Institute conducting this study expects the quality of AI algorithms to continue to grow. A full description of the experiment was published in JAMA Oncology.
W on a five-point scale. Currently, we are witnessing a significant technological acceleration and reaching the IV level (high automation), when the system independently automatically processes the received data and provides the specialist with pre-analyzed information. This saves time, avoids human error and provides more efficient patient care. That's what he judged a few months ago Stan A.I. in the field of medicine close to him, prof. Janusz Braziewicz from the Polish Society for Nuclear Medicine in a statement to the Polish Press Agency.
4. Machine viewing of medical images
Algorithms, according to such experts as prof. Brazievicheven indispensable in this industry. The reason is the rapid increase in the number of diagnostic imaging tests. Only for the period 2000-2010. the number of MRI examinations and examinations has increased tenfold. Unfortunately, the number of available specialist doctors who could carry them out quickly and reliably has not increased. There is also a shortage of qualified technicians. The implementation of AI-based algorithms saves time and allows full standardization of procedures, as well as avoiding human error and more efficient, personalized treatments for patients.
As it turned out, also forensic Medicine can benefit from development of artificial intelligence. Specialists in this field can determine the exact time of death of the deceased by chemical analysis of the secretions of worms and other creatures that feed on dead tissues. A problem arises when mixtures of secretions from different types of necrophages are included in the analysis. This is where machine learning comes into play. Scientists at the University of Albany have developed an artificial intelligence method that allows faster identification of worm species based on their "chemical fingerprints". The team trained their computer program using mixtures of different combinations of chemical secretions from six species of fly. He deciphered the chemical signatures of insect larvae using mass spectrometry, which identifies chemicals by accurately measuring the ratio of mass to electric charge of an ion.
So, as you can see, however AI as an investigative detective not very good, it can be very useful in a forensic lab. Perhaps we expected too much from her at this stage, anticipating algorithms that would put doctors out of work (5). When we look at Artificial Intelligence more realistically, focusing on specific practical benefits rather than the general, her career in medicine looks very promising again.
5. Vision of the doctor's car
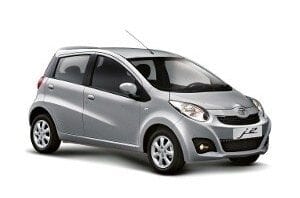
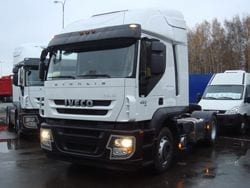